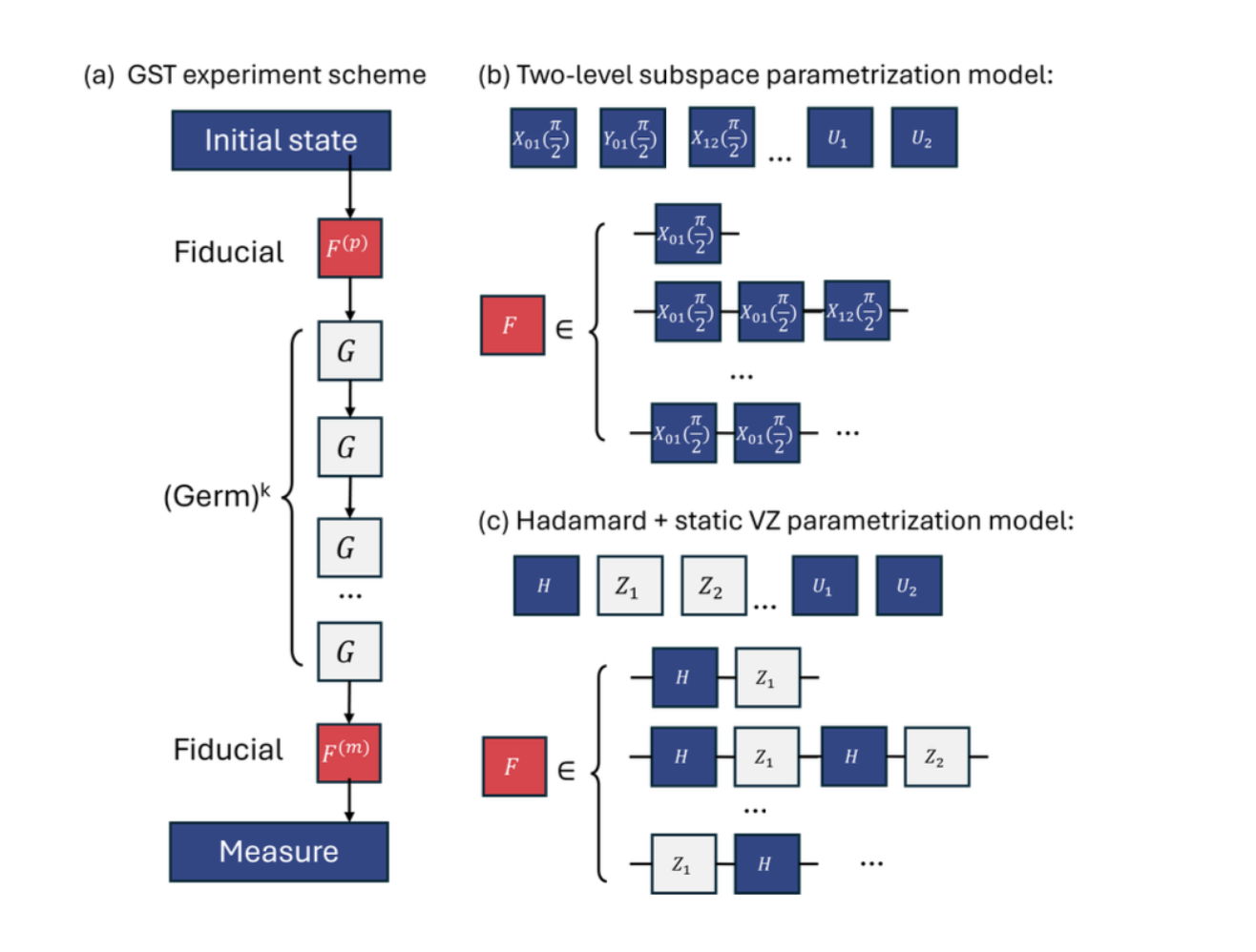
QUICKBITS
Efficient characterization of qudit logical gates with gate set tomography using an error-free Virtual-Z-gate model
- Using this methodology it will be possible to make Gate Set Tomography (GST) more practical for larger quantum systems as it reduces the computational burden while still accurately characterising the systems performance.
- This research extends the GST to qudits based systems.
- These experiments show that you don’t always need to go through the more complicated and time-consuming traditional process to get accurate results. By making a few smart assumptions, you can save a lot of time and resources while still getting the same quality of information.
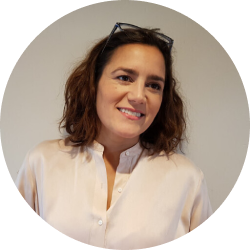
Katy Alexander
MARKETING DIRECTOR
Katy is the Marketing Director at OQC. Prior to joining OQC, she developed and scaled marketing and analytics functions for startups and large listed companies. Passionate about using data to guide strategic decisions, Katy’s unique blend of analytical rigour and creative expression enables her to tackle diverse challenges effectively. In her spare time, she champions diversity in STEM through the creation of games and education resources for primary schools.
This paper, led by a research fellow Dr. Shuxiang Cao from our CSO’s university lab, explores an exciting and new methodology which offers a more efficient way to characterise the performance of quantum systems. Quantum processors are essential for quantum computing, where logical gates (similar to logic gates in classical computers) manipulate qubits or in the case of this paper, qudits.
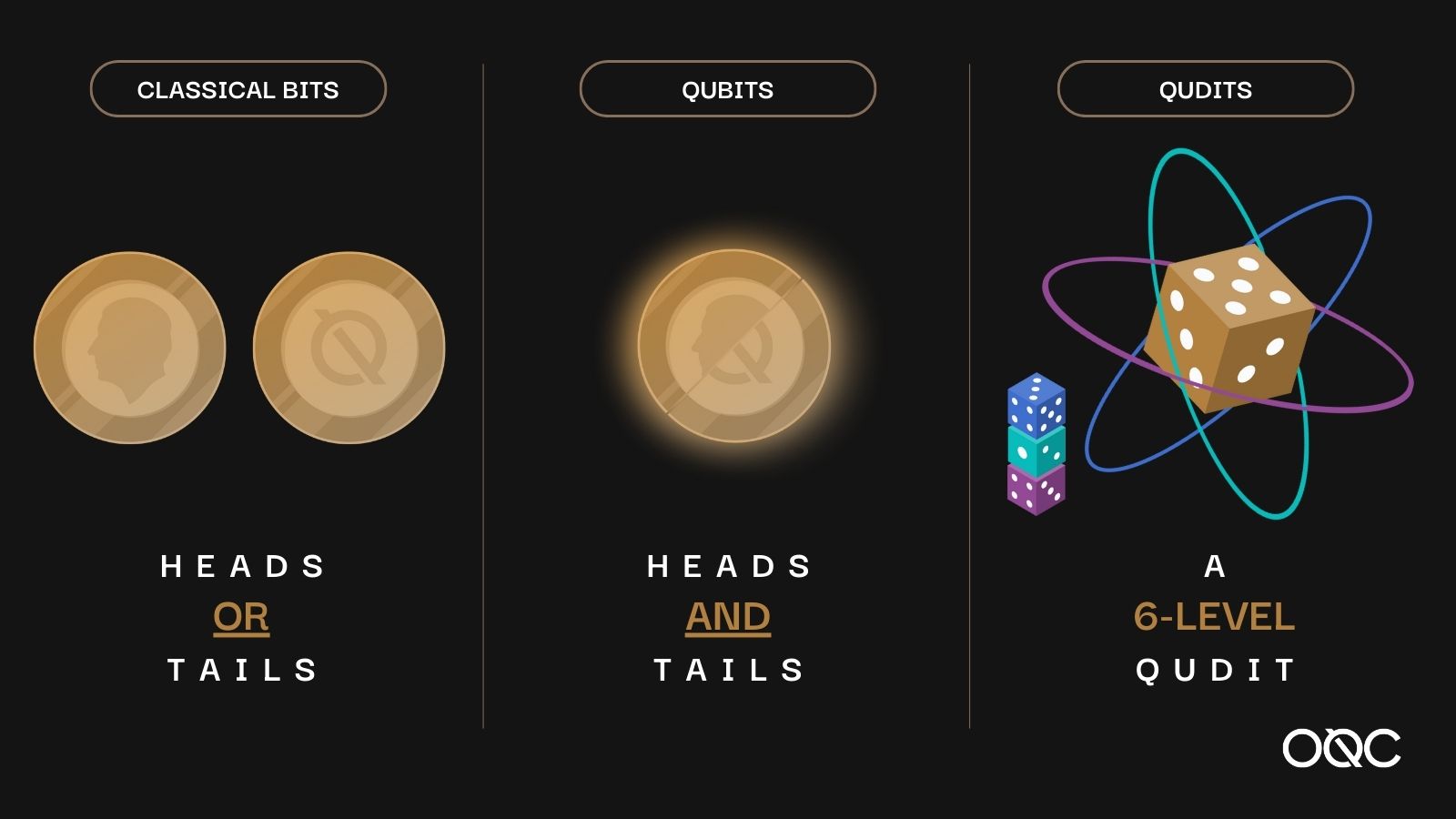
Qudits are a multi-level computational unit. Qudits provide a larger state space to store and process information.
While qubits are two-level systems that can be in state 0 or 1, or a superposition of both; qudits are systems with more than two levels. A qudit is a d-level quantum system where d can be any integer greater than 2. In a qudit, the state space is expanded to include more levels, meaning it can exist in a superposition of d different states, which are typically labelled as |0⟩, |1⟩, …, |d-1⟩.
Qudits could be advantageous because they increase information capacity, they also open the way for quantum algorithms to be more efficiently implemented because qudits can perform more complex operations with fewer gates. This can lead to shorter circuit depths and potentially lower error rates. However, characterising qudits has traditionally been very costly in terms of computational resources.
Snapshot: What is Characterisation?
In the context of quantum computing, characterisation refers to the process of accurately determining the properties, performance, and errors of quantum components, particularly quantum gates, states, and measurements. It is a key step in the development and deployment of quantum systems as it enables the understanding and mitigation of errors, ensuring that quantum systems can operate reliably at scale. The ultimate goal of characterisation is to bring quantum components as close to their ideal performance as possible, paving the way for practical and error-tolerant quantum computing.
A New Methodology
Gate Set Tomography (GST) is a predictive characterisation method of logic operations (gates) on quantum computing processors. In traditional methods, each two-level system (or subspace) within a qudit is parameterised separately, which results in a method that is parameter-heavy and computationally expensive. This has a real impact on the ability to scale systems.
In Figure 2 – see below – the authors have compared the number of parameters required for characterisation using the traditional methodology (marked b in the figure) which requires significantly more parameters, versus their new methodology (marked c in the figure) which drastically reduces the number of parameters required.
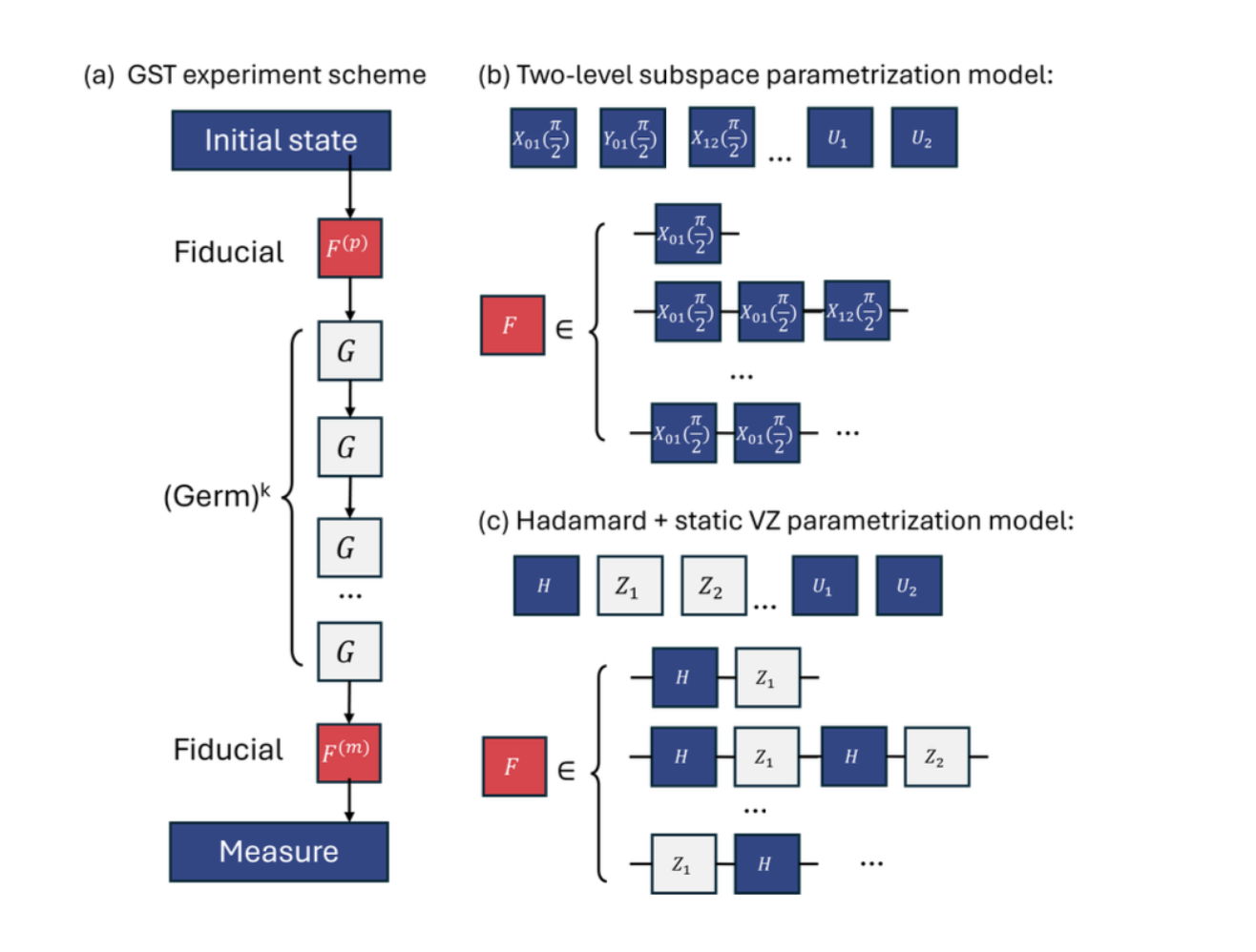
Virtual Z gates are special because they don’t physically alter the qudit’s state but instead change the phase of the operations. By assuming these gates are perfect, the new method simplifies the GST process, reducing computational costs and data collection needs.
The improved method focuses on reducing the number of parameters. In the traditional approach, besides parametrising the gate of interest, a large set of gates in two-level subspaces are also parametrised to ensure the completeness of the method. Instead, the authors have proposed and demonstrated an innovative configuration that requires only the Hadamard gate “H” to be parametrised as overhead. By focusing on this gate alone, they were able to simplify the model significantly.
Experimental Testing
To test their new method, they performed experiments using a special type of quantum system called a “qutrit.” A qutrit is a qudit with three levels instead of two, making it more complex but also more powerful for certain quantum computations.
They compared three different methods for characterising (or measuring) how well the qutrit’s gates work:
- The traditional method doesn’t assume virtual Z gates are special in any way, and therefore the method has to consider the possibility of errors in every part of the system. This takes a lot of time and computing power.
- Alternative methods such as randomised benchmarking (RB), cross entropy benchmarking (XEB) which are simpler and faster but less accurate and often used for rough estimates were also explored.
- Leek Lab’s new method assumes that the virtual Z gates are perfect and error-free. By making this assumption, they drastically reduced the number of measurements and calculations needed, making the whole process faster and easier without sacrificing accuracy.
This new method produced almost identical results to the traditional method, requiring much less effort. This is a strong indication that their assumption about the virtual Z gates being error-free was accurate. This means that Leek Labs new method can be used to accurately characterise more complex quantum systems, like those with multiple qubits or qutrits, without getting bogged down by too many calculations.
Why I’m excited about this article?
These experiments show that you don’t always need to go through the more complicated and time-consuming traditional process to get accurate results. By making a few smart assumptions, you can save a lot of time and resources while still getting the same quality of information. This new GST method successfully characterises qudit logic gates with comparable accuracy to traditional methods but with reduced time and computational resources. This is a promising tool for characterising qudit-based quantum processors, making it easier to develop and scale quantum computing technologies. This approach could also be particularly useful as quantum processors become more complex.