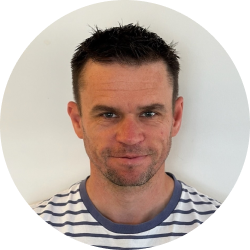
Peter Leek
CHIEF SCIENTIFIC OFFICER
Peter founded OQC in 2017 and is a pioneer in the field of quantum computing, particularly known for his contributions to superconducting quantum circuits. With a PhD from the University of Cambridge, and postdoctoral research experience from ETH Zurich in the science of circuit QED, he has led his own research group at the University of Oxford since 2012, where OQC’s core IP was first developed. Peter is also a co-investigator in the UK’s national quantum computing research hub, where he continues to play a prominent role in the national programme.
EVENT DETAILS
SEEQA is a one week residential event in Oxford’s historic Merton College, where experts will gather to debate the challenges and opportunities in the worldwide effort to achieve general Quantum Advantage.
Key themes and topics of the event will include; early fault tolerance and error mitigation, hybrid algorithms, benchmarking and quantifying progress, ‘Is there QA in Chemistry?’, QC to augment emerging classical methods, randomized protocols and classical shadows, and emulation, and dequantizing algorithms.
Our CSO, Dr. Peter Leek will be in attendance during the week at the SEEQA Conference 2024, which OQC is proud to be a premium sponsor of.
POSTER SESSION AND OQC POSTER PRIZE
We have created a Poster Prize of £500 to award the most outstanding young researcher’s poster. All students (undergraduate and postgraduate) and early career researchers who are within 4 years since completion of their doctorate are eligible to nominate themselves for this prize.
OQC POSTER PRIZE WINNERS
Congratulations to the winners of the poster session at SEEQA 2024.
Martina Nibbi
Poster title: Block encoding of matrix product operators
Summary: Quantum signal processing combined with quantum eigenvalue transformation has recently emerged as a unifying framework for several quantum algorithms. In its standard form, it consists of two separate routines: block encoding, which encodes a Hamiltonian in a larger unitary, and signal processing, which achieves an almost arbitrary polynomial transformation of such a Hamiltonian using rotation gates. The bottleneck of the entire operation is typically constituted by block encoding and, in recent years, several problem-specific techniques have been introduced to overcome this problem. Within this framework, we present a procedure to block-encode a Hamiltonian based on its matrix product operator (MPO) representation. More specifically, we encode every MPO tensor in a larger unitary of dimension D+2, where D = ⌈log(χ)⌉ is the number of subsequently contracted qubits that scales logarithmically with the virtual bond dimension χ. Given any system of size L, our method requires L+D ancillary qubits in total, while the number of one- and two-qubit gates decomposing the block encoding circuit scales as O(L * χ^2).
Read the full article on arXiv.
Sofiene Jerbi
Poster title: Shadows of quantum machine learning
Summary: Quantum machine learning is often highlighted as one of the most promising practical applications for which quantum computers could provide a computational advantage. However, a major obstacle to the widespread use of quantum machine learning models in practice is that these models, even once trained, still require access to a quantum computer in order to be evaluated on new data. To solve this issue, we introduce a class of quantum models where quantum resources are only required during training, while the deployment of the trained model is classical. Specifically, the training phase of our models ends with the generation of a ‘shadow model’ from which the classical deployment becomes possible. We prove that: (i) this class of models is universal for classically-deployed quantum machine learning; (ii) it does have restricted learning capacities compared to ‘fully quantum’ models, but nonetheless (iii) it achieves a provable learning advantage over fully classical learners, contingent on widely believed assumptions in complexity theory. These results provide compelling evidence that quantum machine learning can confer learning advantages across a substantially broader range of scenarios, where quantum computers are exclusively employed during the training phase. By enabling classical deployment, our approach facilitates the implementation of quantum machine learning models in various practical contexts.
Read the full article on nature.